With the backdrop of science’s replication and now credibility crisis, triggered perhaps by psychology but now much broader than that, I/O psychology is finally beginning to reflect. This is great news. Deniz Ones and colleagues recently published a paper in our field’s professional journal, the Industrial-Organizational Psychologist (TIP), providing some perspective on the unique version of this crisis that I/O now faces, a crisis not only of external credibility but also of internal credibility. In short, I/O practitioners and the world more broadly increasingly do not believe that I/O academics have much useful to say or contribute to real workplaces. Ones and colleagues outline six indicators/causes of this crisis:
- an overemphasis on theory
- a proliferation of, and fixation on, trivial methodological minutiae
- a suppression of exploration and a repression of innovation
- an unhealthy obsession with publication while ignoring practical issues
- a tendency to be distracted by fads
- a growing habit of losing real-world influence to other fields.
I will let you read the description of each of these individually in the authors’ own words, but let me just say that I agree with about 95% of their comments and would even go a step further. Our field is just past the front edge of a credibility crisis, one that has been bubbling beneath the surface for the last couple of decades. Things aren’t irreversible yet, but they could be soon. The only valid response at this point is to ask, “what should we do about it?” Ones and colleagues offer an unsatisfying recommendation:
To the old guard, we say: Be inclusive, train and mentor scientist–practitioners. Do not stand in the way of the scientist–practitioner model. To the rising new generation, we say: Learn from both science and practice, and chart your own path of practical science, discovery, and innovation. You can keep I-O psychology alive and relevant. Its future depends on you.
To be clear, I completely agree with this in principle. The training model needs to be changed. Researchers need to follow best path of investigation for their personal scientific research questions. We must integrate science and practice into a coherent whole. But if the replication crisis has taught me anything, it’s that waiting for the old guard to become levers of change is too slow and too damaging to the rest of us to accept. When charting your own path of practical science can lead to denial of tenure, the system is fundamentally broken. So if you want change, you must be the lever. I have tried to be such a lever, in my own small way, since I earned my PhD eight years ago. But I am eager for more to join me.
Few of you know this, but I named this blog “NeoAcademic” when I graduated in 2009 not only because I was a newly minted PhD about to set off into academia but also because I was already frustrated then with “the way we do things.” There seemed to be arbitrary “rules” for publishing in top journals that had relatively little to do with “making a contribution,” at least the way I understood the traditional meaning of the word, “contribution.” I heard grumblings from my adviser and the remainder of the Minnesota faculty about how publishing expectations for the Journal of Applied Psychology were “changing” and not necessarily for the better. But it wasn’t a “crisis.” Not yet. I just thought something was up, although I wasn’t quite sure what it was, at the time.
Focused on my own path to tenure, I saw technology as a pressing need for I/O psychology, so I naively decided to innovate through research, hearkening back to the I/O psychologists of old that actually discovered things useful to the world of work. That is, after all, why I wanted to go into academia – to trail blaze at the forefront of knowledge. As anyone who has done innovative research knows, and something I underappreciated at the time, the risk/reward ratio becomes quite a bit poorer when you innovate through research. Several folks here told me I was crazy for trying such a thing, especially pre-tenure. Why would you spend two years investigating a hypothesis that might not turn out to be statistically significant? Because it’s important to discover the answer, regardless of what happens; that’s why.
Unfortunately, I soon discovered that our top journals did (and do) not particularly appreciate this sort of approach. True innovation, when you operate at the boundaries of what is known, is messy and mixes inductive and deductive elements. It involves the creation of new paradigms, new methodologies, and operating in a space in the research literature that is not well explored. As anyone who has published in Journal of Applied Psychology knows, that’s not “the formula” that will get you published there. True innovation is treated a “nice to have,” not a driving reason to conduct research. That felt backwards to me. It felt like our journals were publishing information that was of relatively dubious worth to any real, live human being other than other people trying to publish in those journals. It was definitely not a path toward building a “practical science.” So with that thought, still pre-tenure, I found myself a bit lost.
My initial reaction to this was to think that business schools would save me, that surely business schools would be focused on helping businesses and not just writing papers that other academics will read. I even joined the executive committee of the Organizational Behavior division of the Academy of Management as a way to expose myself to that side of the “organizational sciences.” Unfortunately, I discovered that the problem there is even worse than it is here. For example, most business schools actually name a short list of “A journals” that one must publish in to be tenured and/or promoted. That seemed absolutely backwards to me. It the very worst possible realization of goal setting. It stifles innovation and limits the real world impact of our work. It explicitly creates an “in club,” establishing pedigree as the most important driver of high quality publishing instead of scientific merit. And perhaps worst of all, it encourages people to fudge their results, or at least to take desirable forking paths, for fame and glory. This sort of thinking is now deeply ingrained in much of Academy; it has become cultural.
So with that observation, I decided business schools were a cure worse than the disease, at least for me. I would find little practical, useful science there, at least in OB. (To be fair, when I raised this issue with an HR colleague, they just rolled their eyes at me and said, “Yeah, well, that’s OB.”) In response to this discovery, I turned to colleagues in disciplines outside of both psychology and the organizational sciences. Still later, I turned to interdisciplinary publication outlets. My eyes were opened.
In short, if all you know is I/O psychology, you have an incredibly narrow and limited view of both academic research and the organizational context in which we attempt to operate. I/O psychology is tiny. It often doesn’t feel that way when you’re sitting at a plenary among the thousands at SIOP, or even at Academy, but the ratio of I/O psychologists to the number of people practicing HR related tasks in the world related to what we do is essentially zero. We are a small voice in one corner of one room of a very large building. I/O psychologists often complain that we don’t get a “seat at the big table,” a voice on the world or even national stage, but that’s essentially impossible to do when we won’t let anyone anyone sit at ours either by imposing strange and arbitrary rules on “the way things are done.” We’ve become a clique, and that’s dangerous.
Just like business schools, I/O psychologists use an intense focus on methodological rigor and “rigorous” theory as a way to exclude people who don’t have the “right” training. I recall vividly a time when I once described the expectations for publication in I/O journals to a computer science colleague. I told him offhandedly that a paper could be rejected because its scales were not sufficiently validated in prior studies. He literally laughed in my face. What about situations where previously validated scales were unavailable, or perhaps a situation where scale length was a concern? What if the scale just didn’t work out like you’d expected, despite good reason to trust it? What if data were available in a context where they usually were not? Apparently no study at all is better than one with an alpha = .6.
Now, the bright side. Unlike business schools, I/O psychology now graduates a huge number of Master’s and Ph.D. practitioners who see academia’s sleight of hand for what it really is and then call us out on it. Yet what many academic I/Os still don’t realize, I think, is that many of our students go into practice not because they can’t “hack it” in academia, but because they see the hoops and hurdles to getting anything published, which is presented as the sole coin of the realm, as unpleasant, unnecessary, and lacking or at least diminishing any impact of that research on the real world. This growing chorus of voices, I think, is our field’s saving grace – if only we listen and change in response.
That brings me all the way back around the core question I asked at the beginning of this article: what do we do now? The short answer is 1) change your behavior and 2) get loud. Here are some recommendations.
- If your paper is rejected from a journal because you purposefully and knowingly traded away some internal validity for external validity, complain loudly. If the only way you can reasonably address a research question is by looking at correlational data inside a single convenient organization, the editor should not use criticisms of your sampling strategy as a reason to reject your paper. If Mechanical Turk is the best way, same thing. Do not let journal gatekeepers get away with applying their own personal morality to your paper. Many (although not all) of these people in positions of power are part of the “old guard” because they exploited the system themselves and expect those that follow behind them to do the same. You need to let them know that this sort of rejection is unacceptable. Do not do this with the expectation or even hope that you will get your manuscript un-rejected. Do this because it’s right. Change one mind at a time.
- Prioritize submissions to journals that engage in ethical editorial practices. Even better, submit to these journals exclusively. Journals live and die by citation and attention. If you ignore journals engaging in poor practices, they will either adapt or disappear. I’ve talked about this elsewhere on this blog, suggesting some specific journals you should pay attention to, but this list is growing. Can you imagine the effect of a journal with an 8% acceptance rate suddenly ballooning to 30% because so many people stop submitting there? Like night and day.
- Casually engage in the broader online community. There are many social media sites now devoted to discussing the failings of psychology, and the credibility crisis broadly, and what to do about these problems. Be a part of these discussions. Don’t let other fields, other people or the news media dominate these conversations. IO psychology is strangely quiet online, limited to Reddit, a handful of Facebook and LinkedIn groups, and an even smaller number of blogs. In the modern era, this lack of representation diminishes our influence. Speak up. You don’t need to be sharing the results of a research study to have something worthwhile to say.
- It’s time for some difficult self-reflection: Is your research useless? In another TIP article this issue, Alyssa Perez and colleagues went through the “top 15” journals in I/O psychology, concluding that of 955 articles published in 2016, only 47 reached standards they would consider potentially having “significant practical utility.” Would your publications be one of the 47 or one of the 908? Importantly, Perez just developed some potential criteria for making a utility judgment, and perhaps quite stringent ones, but I would call your attention to these questions to ask yourself of each of your papers, derived from their list. If you answer “no” to any of these, it’s time for a change. And if you think any of these aren’t worthwhile goals, you’re part of the problem:
- Does it tackle a pressing problem in organizations, either directly or indirectly, including but not limited to SIOP’s list of Top 10 Workplace Trends?
- Can the results of the study be applied across multiple types of workplace settings (as opposed to only advancing theoretical understanding)?
- Are the effect sizes of the study both significant and practically meaningful?
- Share your research and perspective beyond the borders of I/O psychology. Research is not the sole domain of academics; we are all scientist-practitioners, and we all have a shared responsibility to conduct research and spread it not only among each other but also among all decision-makers in the world. And if “the world” is scary, feel free to start with “HR.” Don’t hold your nose while you’re over there. Share your passion for scientific rigor and engage in good faith – because such rigor is truly a rare and significant strength of our field – but don’t use that expertise as a tool to brush aside the hoi palloi.
- Innovate by seeking out what I/O-adjacent fields are doing and learn all about them as if you were in graduate school again (or still are). This is a personal pet peeve of mine; a lot of I/O psychologists, both practitioner and academic, want to operate in a bubble. They want to apply their own little sphere of knowledge, whether it’s what they learned in grad school or what they took that workshop on once, use that and be done. It’s not that easy anymore. It shouldn’t be. Self-directed learning is becoming increasingly important as the speed of innovation increases in the tech sector. Many bread-and-butter I/O psychologists can already be replaced with algorithms, and this will only get worse in the coming years. Make sure you have a skill set that can’t be automated, and if you’re at risk of being automated, go get more skill sets. I’m trying to do my part over at TIP, but this is ultimately something I/Os need to do for themselves. If you need motivation, imagine the day when an algorithm can develop, administer, and psychometrically validate its own scales and simulations. That day is coming sooner than you think.
- Accept that sometimes, engineering can be more important than science. When doing science, we aim to use the experiences and circumstances of a single sample, or group of samples, to draw conclusions about the state of the world. When doing engineering, we try to use science to solve a specific problem for one population that is staring us in the face. I/O practitioners, most often, are engineering. They are using the research literature and their own judgment to create solutions to specific problems that specific organizations are facing. These are learning opportunities, all. They need to have a place in our literature and conversations. They are also the primary avenue by which fields outside of I/O learn about who we are and what we do. You must actively try to be both a scientist of psychology and an engineer of organizational solutions. And when your engineering project is successful, remember to trumpet the value of I/O psychology for getting you there.
Ultimately, I think these actions and others like them are not only the way to make academic I/O psychology relevant again but also the way to distinguish ourselves from business schools. Let them have their hundred boxes and arrows, four-way interactions, and tiny effects sizes. Leave solving the real problems to I/O psychology.
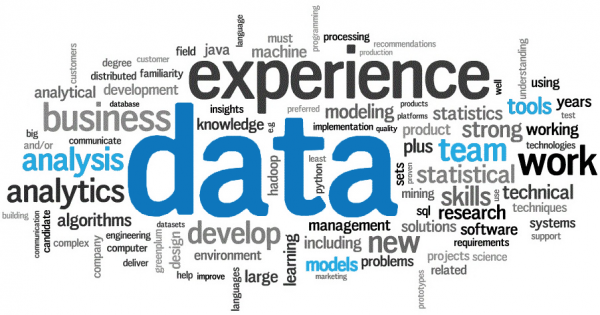
Image by Arpit Agrawal
Are you a psychologist interested in learning some new techniques to leverage data science in your academic research or in your consulting practices? Web scraping may be the answer you need.
Last year, I published the first in what will likely be a series of articles focused on teaching psychologists techniques from data science. Specifically, I introduced the concept of web scraping, which involves the systematic, algorithmic curation of unstructured online data, usually from social media, and its conversion into an analyzable dataset. I furthermore provided a step-by-step tutorial explaining how to use the free programming language Python and its free package scrapy to do just that.
This year, I’ll be presenting three workshops on web scraping in various venues, although these presentations will be in R. Each presentation is somewhat different in focus and learning objectives, so feel free to attend all three!
- April 28, 2017: Automated conversion of social media into data: Demonstration and tutorial (3 hours)
Part of the Friday seminar series at the 2017 Annual Conference of the Society for Industrial and Organizational Psychology (SIOP) in Orlando, FL. - July 17, 2017: Web scraping and machine learning for employee recruitment and selection: A hands-on introduction (3.5 hours)
A pre-conference workshop for the International Personnel Assessment Council (IPAC) annual conference in Birmingham, AL. - August 3-6 (TBD), 2017: How to create a dataset from Twitter or Facebook: Theory and demonstration (1.8 hours)
A skill-building session for the American Psychological Association (APA) annual conference in Washington, DC.
All three presentations will start with an explanation of data source theories, the key theoretical consideration that affects external validity when trying to identify high quality sources of online information for research.
Additionally, the SIOP presentation will focus on instruction in R and rvest, mimicking the online tutorial I provided but with some extra information and a lot of hands-on examples.
The IPAC presentation will focus on the practicals of web scraping, including discussion of tradeoffs to various data sources when using web scraping for employee selection and recruitment, demonstration of both easy-to-use commercial scraping packages and the manual, R-based approach, and interactive discussion of use cases.
The APA presentation will be a hands-on walkthrough of accessing the Facebook and Twitter APIs to web scrape without nearly as much programming as you need when you don’t have an API!
With any of the three, you should be able to leave the workshop and curate a new internet-sourced dataset immediately!
I believe all three provide CE credit, but I’ll update this when I know for sure! See you in Orlando, Birmingham and Washington!
Landers, R. N. (2020). Calculating LongString in Excel to detect careless responders. Authorea. DOI: 10.22541/au.160590023.35753324/v1
Careless responding is one of the most fundamental challenges of survey research. We need our respondents to respond honestly and with effort, but when they don’t, we need to be able to detect and remove them from our datasets. A few years ago, Meade and Craig1 published an article in Psychological Methods exploring a significant number of techniques for doing exactly this, ultimately recommending a combination of three detection techniques for rigorous data cleaning, which, let’s face it, is a necessary step when analyzing any internet survey. These techniques are even-odd consistency, maximum longstring, and Mahalanobis D:
- The even-odd consistency index involves calculating the subscale means for each measure on your survey, split by even and odd items. For example, the mean of items 1, 3, 5, and 7 would become one subscale whereas the mean of items 2, 4, 6, and 8 would become the other. Next, you take all of the even subscales and pair them with all of the odd subscales across all of the measures in your survey, calculate a correlation, and then apply the Spearman-Brown prophecy formula to adjust the value up to a scale of -1 to 1.
- Maximum LongString is the largest value for LongString across all scales on your survey, where LongString is the number of identical response in a row. Meade and Craig recommended LongString would be most useful when the items were randomly ordered.
- Mahalnobis D is calculated from the regression of scale means onto all the scores that inform them. In a sense, you are trying to see if responses to individual items correspond with the scale mean they created consistently across individuals. Some conceptualizations of this index regress participant number onto scores, which conceptually accomplishes basically the same thing.
In all three cases, the next step is to create a histogram of the values and see if you see any outliers.
Calculating Careless Responding Indices
Of these three, Mahalanobis D is the most easily calculated, because saving Mahalanobis D values is a core feature in regression toolkits. It is done easily in SPSS, SAS, R, etc.
The second, the even-odd consistency index, is a bit harder but still fundamentally not too tricky; you just need to really understand how your statistical software works. Each step, individually, is simple: calculate scale means, calculate a correlation, apply a formula.
The third, Max LongString, is the most intuitively understandable but also, often unexpectedly, the most difficult to calculate. I imagine that the non-technically-inclined generally count by hand – “this person has a maximum of 5 identical answers in a row, the next person has 3…”
An SPSS macro already exists to do this, although it’s not terribly intuitive. You need to manually change pieces of the code in order to customize the function to your own data.
Given that, I decided to port the SPSS macro into Excel and make it a little easier to use.
An Excel Macro to Calculate LongString
Function LongString(cells As Range) Dim cell As Range Dim run As Integer Dim firstrow As Boolean Dim maxrun As Integer Dim lastvalue As String firstrow = True run = 1 maxrun = 1 For Each cell In cells If firstrow = True Then firstrow = False lastvalue = cell.Value Else If cell.Value = lastvalue Then run = run + 1 maxrun = Application.Max(run, maxrun) Else run = 1 End If lastvalue = cell.Value End If Next cell LongString = maxrun End Function
To Use This Code Yourself
- With Excel open, press Alt+F11 to open the VBA Editor.
- Copy/paste the code block above into the VBA Editor. In newer versions of Excel, you may not see a code window open by default. If that happens, right-click on VBAProject for the workbook you want to add the macro to, click Insert, and then Module. This should open a blank text window called “Module1” where you can paste the code block.
- Close the VBA Editor (return to Excel).
- In an empty cell, simply type =LONGSTRING() and put the cell range of your scale’s survey items inside. For example, if your first scale was between B2 and G2, you’d use =LONGSTRING(B2:G2)
- Repeat this for each scale you’ve used. For example, if you measured five personality dimensions, you’d have five longstrings calculated.
- Finally, in a new cell use the =MAX() function to determine the largest of that set. For example, if you put your five LongStrings in H2 to L2, you’d use =MAX(H2:L2)
That’s it! Importantly, the cells needs to be in Excel in the order they were administered. If you used randomly ordered items, this adds an additional layer of complexity, because you’ll need to recreate the original order for each participant first before you can apply LongString. That takes a bit of Excel magic, but if you need to do this, I recommend you read up on =INDIRECT() and =OFFSET(), which will help you get that original order back, assuming you saved that item order somewhere.
Final Steps
Once you have Max LongString calculated for each participant, create a histogram of those values to see if any strange outliers appear. If you see clear outliers (i.e., a cluster of very high Max LongString values off by itself, away from the main distribution), congratulations, because it’s obvious which cases you should drop. If you don’t see clear outliers, then it’s probably safer to ignore LongString for this analysis.
Another potential issue with Max LongString is that if you have scales with varying numbers of items, shorter scales can be lost when you calculate the Max. To avoid that problem, try converting each of your longstrings into a proportion. For example, for a 4-item scale, try =LONGSTRING(H4:H7)/4 and then calculate MAX() on those results.